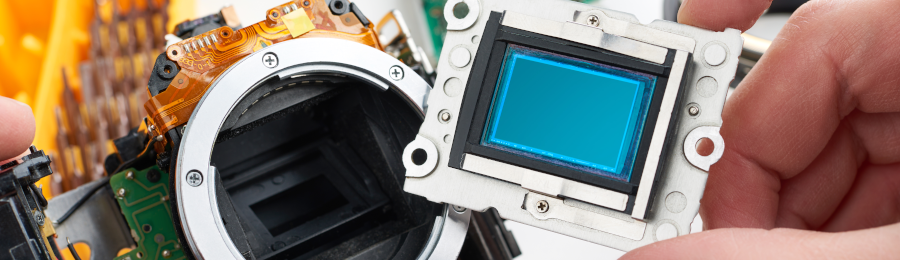
Takes place only in the summer semester
Introduction to compressive sensing and learning (ICS)
Study programs:
Mandatory course for Master Elektrotechnik, Master Informationstechnologie, Master Wirtschaftsingenieurwesen Informationstechnik und Digitalisierung
- Module number: FBE0093 Mehrdimensionale Signale und Systeme (temporary allocation)
Learning outcomes / competencies:
Successful participation in the modules Mathematics A, B.
The students have basic math knowledge, specifically, of linear algebra. Knowledge of probability theory and estimation theory are and advantage. The students will earn the capability of advantageously applying Compressive Sensing theory to develop and understand compressive sensing detection approaches and sparse reconstruction algorithms for the most common signals in engineering.
Contents:
The lecture starts with an introduction to Compressive Sensing. Then the connection between the general sensing setting and compressive sensing is made clear. The connection between the null space of a matrix and the set of possible solutions is shown. The triangular inequalities. Cauchy-Schwartz inequality. Induced matrix norms. Optimality and reconstruction. Relation between uniqueness and recoverability. What is the best s-term approximation of a signal. Importance of the RIP constant for successful ℓ1-minimization. Connection between coherence and RIP. Coherence limits and Geršgorin Disc theorem. Mutual coherence of two unitary bases. Importance of incoherence in sparse signal sampling.
Learning objective:
You will learn the basics of Compressive Sensing, what a sparse signal means, ubiquity of sparse and compressible signals, and how to utilize sparsity in sensing. Students will also learn about the trade-offs in reducing the number of samples and how to reconstruct the sparse signal from the samples.
Where do you need these skills in our research activities?